A Low Code Solution for a Leading Adhesive Manufacturer
Case Study
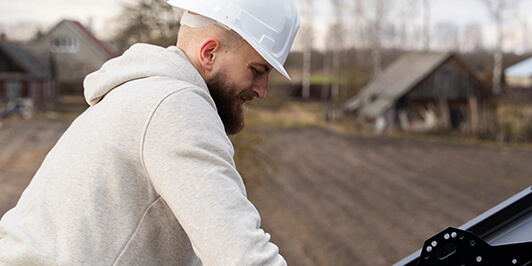
About the Client
A leading adhesive manufacturer that has been a driving force in the industry. Renowned for various iconic brands, the company’s diverse portfolio extends to construction chemicals, art materials, and more. Their commitment to quality and customer satisfaction has solidified their position as a trusted partner for various industries. With a legacy of resilience and a focus on sustainable growth, the company continues to shape the landscape of adhesives, contributing to the success of big and small projects across the region and beyond.
Business Context
Traditionally, manual inspections by trained professionals have been the primary method for identifying defects like cracks and leaks on the damaged surface. However, this approach is often time-consuming, resource-intensive, and prone to human error, leading to potential safety hazards and costly repair delays for the end users of the client’s services. The client was in search of an alternative solution that utilizes AI technology.
Challenge:
The main challenge was to build an automated and scalable solution while keeping the cost in check. The solution was expected to detect 13+ different types of defects. Thousands of users running checks for all different types of defects 24×7 would have led to heavy usage of cloud resources, resulting in high running costs. Thus, the deployed model was expected to execute only when there is an API hit during a specific time period of the day (business hours).
Opportunity:
Considering GCP’s Machine Learning capabilities, Niveus recommended building an automated defect detection and scalable solution on GCP to identify defects on various surfaces, including cracks in parapet walls, leakage, and surface type. We proposed a low code (AutoML) solution to eliminate the need for domain knowledge and specific skills to identify the defects.
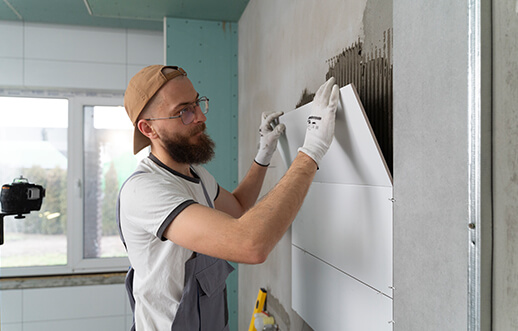
The Solution
Niveus utilized AutoML Image multi-label classification to develop a user-friendly, low-code solution for creating and deploying multi-label image classification models within the comprehensive Vertex AI platform. This AutoML service was part of GCP’s Vertex AI platform, an end-to-end ML platform used to deploy the AutoML models on the endpoints. Automated defect detection is used to discover defects on the surface without the need for an expert, allowing the client to propose corrective actions and solutions quickly and prevent potential hazards to the end user.
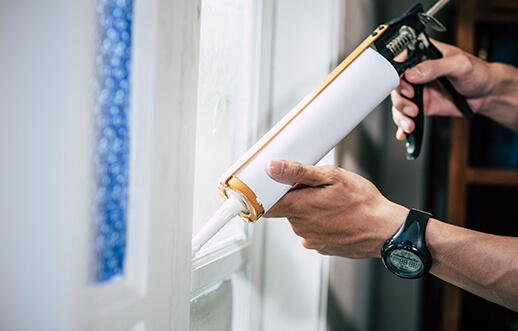
- The images shared by the client were categorized into specific defect categories based on criteria such as defect location, color, texture, and physical characteristics
- Folders were named descriptively to organize images by defect type, and images with multiple defects were placed in corresponding folders
- The categorized image files were zipped and uploaded to the designated cloud storage buckets
- In the Vertex AI UI Console, defect labeling/annotation was performed
- Models were trained to evaluate their ability to detect defects
- Datasets from different categories were merged and regrouped to train and assess combined models, encompassing a broader range of defect types
- Vertex AI/AutoML enabled feature engineering, dataset lineage tracking, training iterations, model generation, hyperparameter tuning, and deployment management
- Cloud Run was used for model deployment
- Bigquery is used to store the model responses, and Cloud Storage is used to save image dataset
Key Value Delivered
- By leveraging the solution, the client could accelerate the defect detection process, enhance service quality, and optimize resource utilization while keeping costs in check
- Early detection helps reduce repair costs for the end user of the client’s service
- The previously manual processes have been fully automated, resulting in significant time and effort savings
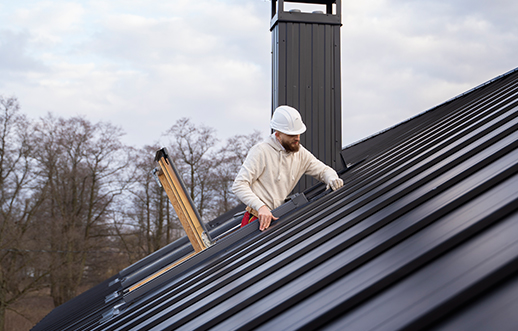
Business Impact
93%
Model Accuracy rate