Case Study
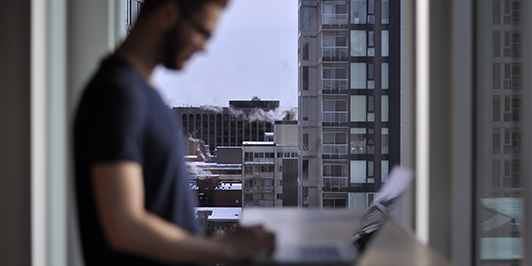
The Client
The client is an Indian subscription-based OTT, video-on-demand entertainment and media platform, launched in 2012. The network offers media streaming and video-on-demand services. The digital platform is accessible on most Internet-connected screens including mobile, tablets, web, and TV. The client broadcasts its contents in different countries to audiences with various languages and backgrounds.
Project Objective
As the client provides entertainment across borders and in multiple countries, language can be considered a hurdle in delivering meaningful content. They are currently manually translating their movies in different languages with the use of subtitles and were looking to automate this process. The first of its kind in the media industry in India, the automation is intended to make translation process simpler and faster, and allow the client to be the first in the market to implement it.
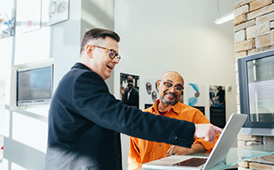
Business Solution
As a part of this engagement, the team from Niveus Solutions has been working closely with the client team to implement AutoML translation of English text into 4 languages. The client ran a POC with Google and Niveus to test the accuracy of the model and the results were convincing to take the workload to production. This is an ongoing engagement on a pay-as-you-go basis where the client will avail Google and Niveus’ services to implement the AutoML translation model for Arabic and other languages. Our subtitle translation services broaden client’s reach to newer regions and audience pools beyond language barriers.
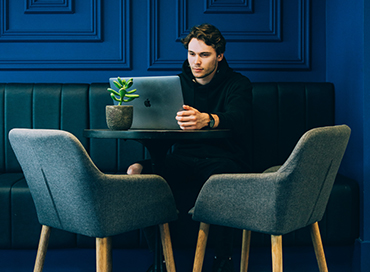
The Impact
Around 7.45 hrs of effort saving per movie
4.84 BLEU Score improvement compared to NMT model after translation
Project to be implemented for 4 languages, 2K movies in each language in the next phase
Implementations
- Identify initial datasets of 100 movies to be validated and used for the production environment and would be scaled up to 2000 movies.
- Create a Dataset with the translation samples available
- Upload the training data
- Data will be in cloud bucket in the form of a CSV file
- Specify the Source (English) and Target Languages
- Choose a base model (NMT)
- Start Training the Model
- Apply glossary and post-translation scripts
- Check the accuracy with the Test data
Low application latency
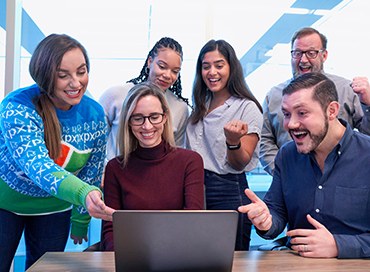