Case Study
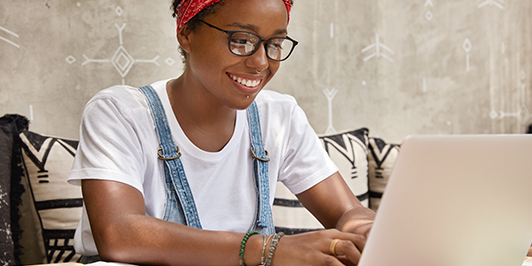
The Client
The client is a digital native enterprise providing a specialized cash accounting application for small businesses in India. Their digital record-keeping app allows users to add entries, segregate records and find overall balance instantly. Their main goal comprises helping small and medium organizations to digitize their business records.
Project Objective
Business Value: Niveus helped the client to streamline customer experience, improve the quality of their services, and reduce TAT on identifying worthwhile interactions with power users.
The client has a variety of users, some of which are inactive/irregular accounts. They wanted to identify their power users who typically record more than 200 entries in the application in a span of 30 days, post a few days of signup. While using manual processes, this app takes about 2 weeks to identify power users. By measuring user engagement and identifying these users, the company was looking to target them with specific marketing campaigns and offers that would result in increased sales. This was expected to help the company to grow its customer base and revenues.
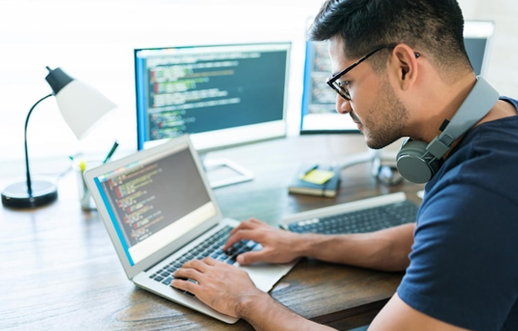
Business Solution
Niveus helped the client with faster identification of power users, which enabled the company to upsell/cross-sell other products and services, reducing their time consumption and boosting their business. Additionally, this data would also be used for targeted marketing to acquire new power users. By measuring user engagement for their digital expense tracking app, the business gained a bird’s eye view on their prime users and their transactions.
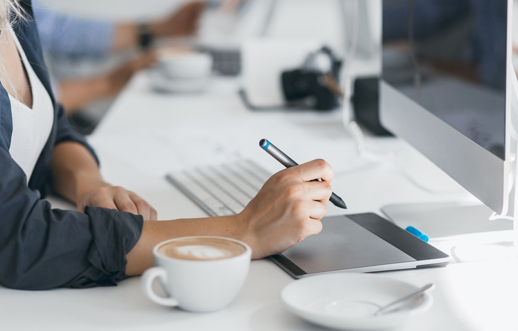
Implementation
- The transactional and online behavior tables are inclusive of data features such as transactions, books, users, invitations, and Google Analytics already present in the client’s BigQuery project
- These tables are modeled into a single table, containing raw and derived features, and the target users for ML modeling
- The BigQuery tables was imported into Vertex AI workbench and Jupyter notebook for exploratory data analysis
- BigQuery ML was used for Classification Model development and its SQL functions can be used to train the model
- Batch prediction can be viewed in the BigQuery ML console. Or else, the model can also be exported to Cloud Storage for managed deployment into Vertex AI’s online prediction
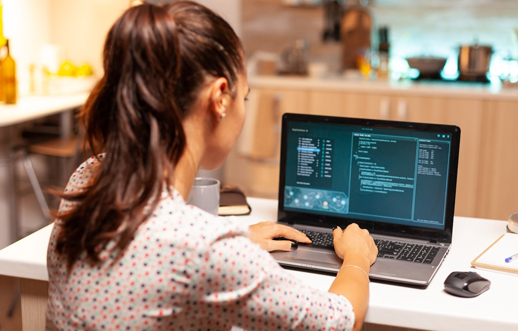