Automated Defect Detection with Image Processing using AI Vision
Asian Paints
93%
model accuracy rate
150+
paint-related defects detected
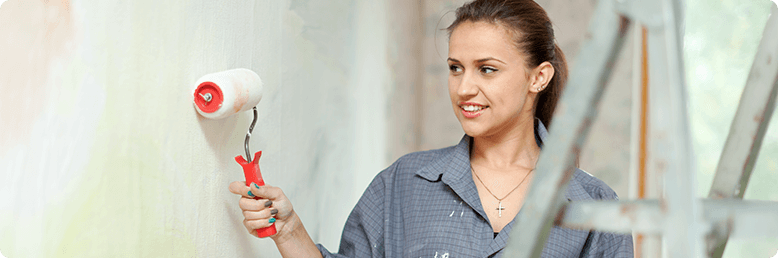
Business Opportunity
Defect Detector with AI/ML Capabilities
Asian Paints, founded in 1942 and headquartered in Mumbai, stands as a global leader and India’s largest paint company, with operations spanning over 60 countries. The company offers a diverse range of home improvement products, including four types of interior wall finishes and a palette of over 2,200 colors, catering to varied design preferences. Beyond paints, their portfolio includes wall coverings, waterproofing solutions, adhesives, and other services.
Traditionally, internal and external defect detection relied on manual inspections by skilled professionals. However, this approach proved to be time-consuming, resource-heavy, and prone to errors, often resulting in delays in customer service. To overcome these challenges, Asian Paints sought to optimize this process and enhance delivery timelines.
Niveus developed a no-code AI solution on Google Cloud Platform (GCP) to precisely detect internal and external defects based on parameters like position, color, texture, and types like algae, blisters, and peeling. The solution ensured comprehensive coverage, streamlining the process and accelerating the service.
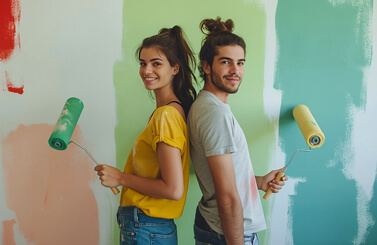
The Challenge
There were 3 key challenges in implementation, namely –
- Cost-Effective Automation: Developing a scalable solution to accurately detect over 15 defect types without excessive expenditure
- Optimized Resource Utilization: Minimizing cloud resource consumption by avoiding simultaneous checks for all defect types.
- On-Demand Model Activation: Ensuring the deployed model operates only during specific time windows triggered by API requests.
Our Solution
Niveus developed an AI-driven solution with Computer Vision and Machine Learning capabilities using Google Cloud Platform (GCP) to help the client visually identify internal and external defects with precision. The no-code platform eliminated the need for domain expertise, offering the flexibility and accuracy required for surface defect detection.
The AI model identified 150+ paint-related defects based on specific criteria, including their position (above, below, or on the surface) and characteristics like color and texture. The solution covered various defect types such as algae, blisters, bubbles, sand particles, peeling, patchiness, and shade fading, ensuring comprehensive defect detection across categories.
GCP’s AutoML was used to build a low-code, multi-label classification model. This approach simplified the process, enabling a single model to detect multiple defect types while maintaining accuracy through confidence threshold clustering. AutoML was chosen for its ability to streamline model development without requiring in-depth machine learning expertise.
Images provided by the client were categorized based on defect types and uploaded to Google Cloud Storage for training. Vertex AI was used to train and annotate the model, ensuring seamless tracking of datasets, models, and deployments. Cloud Run was then utilized to deploy the model as an API, enabling the client to integrate it into their workflows easily.
This solution automated defect detection and provided a scalable and cost-efficient framework for improving inspection processes.
Improved efficiency
Results
- 93% Model Accuracy Rate: Achieved a 93% model accuracy rate for defect detection, resulting in high performance for detecting defects.
- 150+ Paint-Related Defects: Detected over 150 paint-related defect types with precision.
- Improved Efficiency: Automated defect detection reduced inspection time and optimized resource allocation.
- Faster Resolutions: Early defect identification enabled quicker repairs, reducing delays and costs.
Power of Partnership
Niveus brought a comprehensive range of skills and expertise to this implementation, combining advanced knowledge in AI, cloud technologies, and a deep understanding of the client’s specific needs. Our team, consisting of seasoned data scientists, engineers, and project managers, collaborated closely with the client at every stage of the project. We focused on technical execution and ensured that the solution was closely aligned with the client’s business objectives, offering the right balance of scalability, efficiency, and flexibility.
With our experience in implementing complex solutions, we were able to address the unique challenges of defect detection while keeping the client’s long-term goals in mind. Niveus’ ability to deliver high-quality results on time, along with our continuous support, ensured that the solution was successfully integrated into the client’s operations. Our team’s expertise in managing end-to-end project lifecycles, from strategy to deployment, helped facilitate a smooth and impactful transformation, showcasing the true value of collaboration and partnership.
Benefits of Machine Learning Solutions from Niveus
- Increased Automation: Speeds up processes and reduces manual intervention, improving overall efficiency.
- Enhanced Accuracy: Minimizes human error, ensuring more precise and reliable results.
- Scalability and Flexibility: Machine learning adapts to evolving data, allowing solutions to grow with the business.
- Real-Time Insights: Enables instant analysis, facilitating faster decision-making and issue resolution.
- Cost Efficiency: Streamlines workflows, reduces resource waste, and lowers operational costs.
- Proactive Monitoring: Detects potential issues early, preventing costly downtime or delays.

Happy to announce that we have gone live with the Auto-detection of defect functionality. I want to thank all of you for your diligent support of the project and for your sincere efforts. We hope to expand this functionality into more defects in the future and look forward to continuing our partnership.
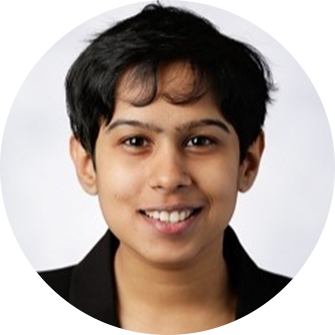
Nandhini N
Systems manager – Asian Paints Ltd.
At a Glance
CLIENT
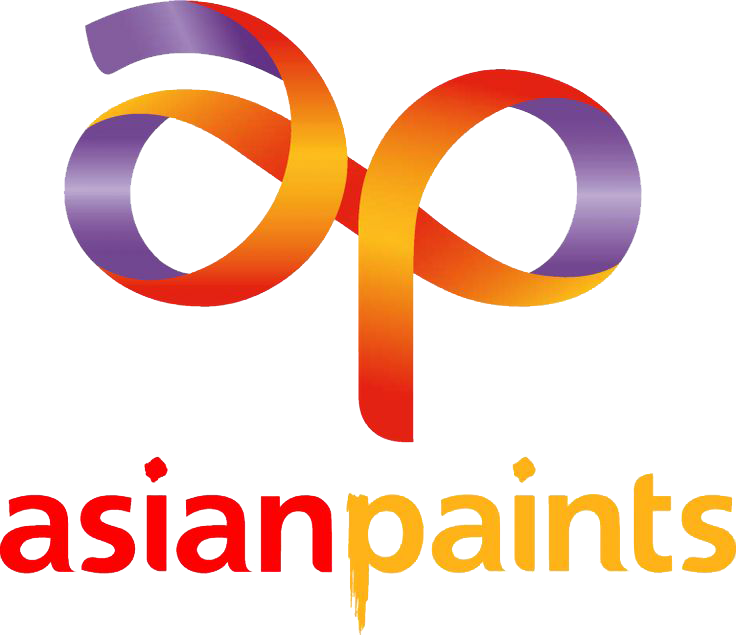
Asian Paints
INDUSTRY
Manufacturing
BUSINESS NEED
Asian Paints aimed to replace time-intensive, error-prone manual defect inspections with a more efficient and reliable solution.
SOLUTION
Niveus built an AI-powered solution on GCP to precisely detect internal and external defects using computer vision and machine learning.
RESULTS
- 93% Model Accuracy rate
- Improved Efficiency
- Faster Resolutions