Case Study
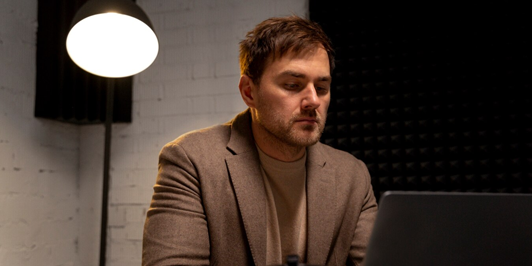
The Client
The client is an online tech-versity for the software enthusiasts of India. They offer an intensive six-month programming course through live classes delivered by tech leaders and subject matter experts. The meticulously structured program enhances the skills of software professionals by offering a modern curriculum with exposure to the latest technologies.
Project Objective – Customer Segmentation Solution
Business Value – By providing a customer segmentation solution, Niveus not only optimized the client’s time and effort, also by providing insights on the reason behind the students’ exiting the course prematurely. Thus helping the client benefit their business in the future.
Based on the students’ engagement behavior/patterns throughout the course, Niveus assisted the client with segmentation of students by providing relevant insight and information on user behavior. This data is then used by the client to facilitate the students in following the course curriculum, and intervening if necessary.
The main objective is to track and monitor events leading to students dropping out of the program. “Not attending class” and “not completing homework” are some of the parameters highlighted which provide insight into the students’ approach towards learning.
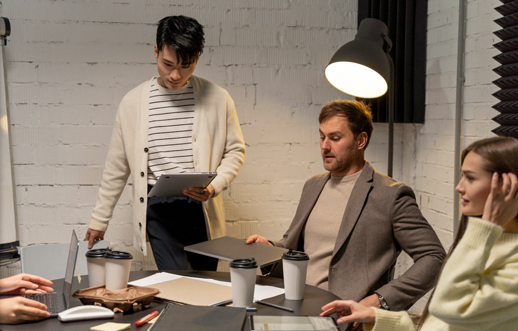
Business Solution
Using the client’s online platform, insights were provided regarding students’ behavior and patterns through the course. This benefited the business by understanding the students’ approach towards the course and helping the client create knowledge-based assignments and make the students’ online learning experience more interactive, useful and comprehensive.
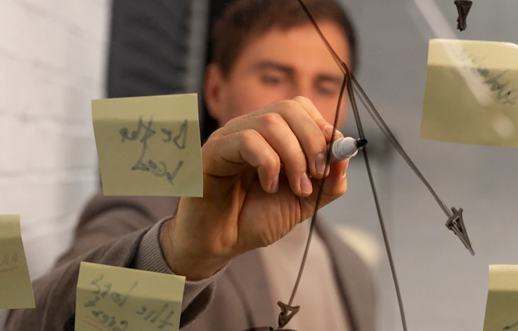
Implementation
- BigQuery Data transfer service is used to transfer data from AWS Redshift to Google BigQuery. A transfer job was created in the data transfer service for the tables that were to be imported.
- Once all the tables are imported into BigQuery Dataset, relevant tables necessary for clustering would be selected to build the ML model
- An Exploratory data analysis is done for the dataset, to examine and analyze the features within the dataset
- All tables are modeled into a single table after determining the clustering field and its relevant attributes
- The BigQuery tables are imported into Vertex AI workbench Jupyter notebook for exploratory data analysis and modeling the table, in addition to using the BigQuery SQL console
- BigQuery ML and its SQL functions are used to train the model and feature engineering
- Batch prediction is viewed in the BigQuery ML console
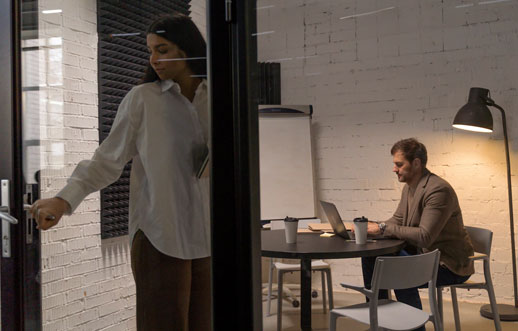