Case Study
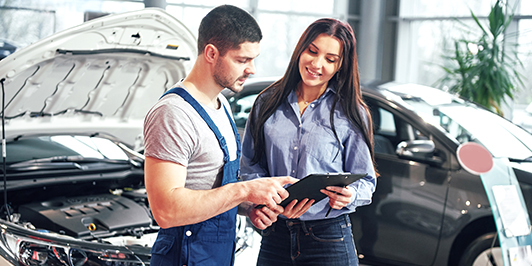
The Client
The client is an Indian multinational automotive manufacturing corporation, and is one of India’s largest conglomerates, spanning across 23 industries and 150+ companies, headquartered in Mumbai. The company has an operational presence in over 100 countries and employs more than 256,000 people. They are well known for their reliable automobiles and tractors, and also for their innovative IT solutions and commitment to rural prosperity.
Project Objective: Data Pipeline Automation
Business Value – The client was looking to address data quality issues in the data that is used for building ML models, using low-code tools.
The customer and product related data from around 7 business units were to be combined into a final master (main) data table through data pipeline automation whose setup is about to be migrated to GCP (BigQuery).
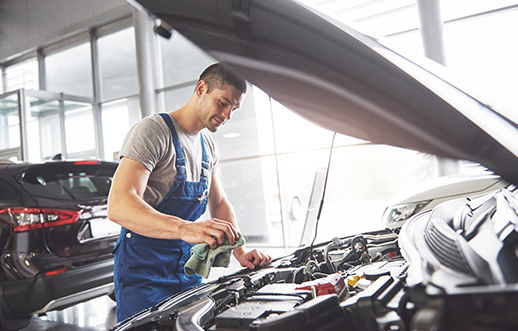
Business Solution
Niveus enabled data pipeline automation, helping the client to combine into a final master data table. It also addressed data quality issues via a custom recommendation ML model across certain business units along with a data quality dashboard with attributes related to the main table.
- The data generated from the main table is transformed into data quality report
- Implementation of the automated DEDUPE data that translates the monthly delta tables into the final data tables for efficient analysis
- Recommendation model that will help build campaigns for enabling cross selling and up selling
- Evaluation of best practices and tools/technologies available in the cloud (GCP) that applies to the data in hand, to build the first version of ML model for recommendation use cases.
- Automation of the ETL pipeline that gets migrated to GCP
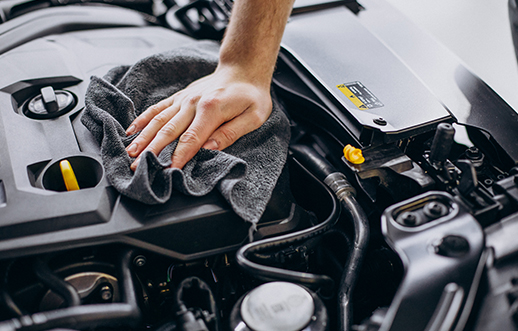
Implementation
- Dataprep is used as a fully managed data service for on-demand scalability to meet the growing data preparation to stay focused on analysis
- The data table undergoes data profiling in terms of the statistical distribution of its various columns. This profiling is enriched with seeking of any data quality requirements including accuracy, completeness, consistency, currency, precision, privacy, etc,.
- The results of the initial profiling and quality rules are triggered for exporting to the BigQuery table via Cloud Storage for dashboarding using Data Studio
- Cloud Dataproc is used to build the custom recommendation model. This helps to create clusters quickly, manage them easily, and save money by turning clusters off when you don’t need them
- Apache Spark is an analytics engine for large-scale data processing
- Cloud Composer is a fully managed workflow orchestration service built on Apache Airflow
- BigQuery ML enables data scientists and data analysts to build and operationalize ML models on planet-scale structured or semi-structured data
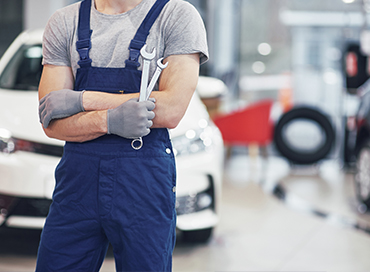